LAPSE:2020.0665
Published Article
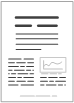
LAPSE:2020.0665
Wind Speed Prediction with Spatio⁻Temporal Correlation: A Deep Learning Approach
June 23, 2020
Wind speed prediction with spatio⁻temporal correlation is among the most challenging tasks in wind speed prediction. In this paper, the problem of predicting wind speed for multiple sites simultaneously is investigated by using spatio⁻temporal correlation. This paper proposes a model for wind speed prediction with spatio⁻temporal correlation, i.e., the predictive deep convolutional neural network (PDCNN). The model is a unified framework, integrating convolutional neural networks (CNNs) and a multi-layer perceptron (MLP). Firstly, the spatial features are extracted by CNNs located at the bottom of the model. Then, the temporal dependencies among these extracted spatial features are captured by the MLP. In this way, the spatial and temporal correlations are captured by PDCNN intrinsically. Finally, PDCNN generates the predicted wind speed by using the learnt spatio⁻temporal correlations. In addition, three error indices are defined to evaluate the prediction accuracy of the model on the wind turbine array. Experiment results on real-world data show that PDCNN can capture the spatio⁻temporal correlation effectively, and it outperforms the conventional machine learning models, including multi-layer perceptron, support vector regressor, decision tree, etc.
Record ID
Keywords
convolutional neural networks, deep learning, Machine Learning, spatio-temporal correlation, wind speed prediction
Subject
Suggested Citation
Zhu Q, Chen J, Zhu L, Duan X, Liu Y. Wind Speed Prediction with Spatio⁻Temporal Correlation: A Deep Learning Approach. (2020). LAPSE:2020.0665
Author Affiliations
Zhu Q: State Key Laboratory of Advanced Electromagnetic Engineering and Technology, Hubei Electric Power Security and High Efficiency Key laboratory, School of Electrical and Electronic Engineering, Huazhong University of Science and Technology, Wuhan 430074, Ch [ORCID]
Chen J: State Key Laboratory of Advanced Electromagnetic Engineering and Technology, Hubei Electric Power Security and High Efficiency Key laboratory, School of Electrical and Electronic Engineering, Huazhong University of Science and Technology, Wuhan 430074, Ch
Zhu L: Department of Electrical Engineering and Computer Science, University of Tennessee, Knoxville, TN 37996, USA
Duan X: State Key Laboratory of Advanced Electromagnetic Engineering and Technology, Hubei Electric Power Security and High Efficiency Key laboratory, School of Electrical and Electronic Engineering, Huazhong University of Science and Technology, Wuhan 430074, Ch
Liu Y: Department of Electrical Engineering and Computer Science, University of Tennessee, Knoxville, TN 37996, USA
[Login] to see author email addresses.
Chen J: State Key Laboratory of Advanced Electromagnetic Engineering and Technology, Hubei Electric Power Security and High Efficiency Key laboratory, School of Electrical and Electronic Engineering, Huazhong University of Science and Technology, Wuhan 430074, Ch
Zhu L: Department of Electrical Engineering and Computer Science, University of Tennessee, Knoxville, TN 37996, USA
Duan X: State Key Laboratory of Advanced Electromagnetic Engineering and Technology, Hubei Electric Power Security and High Efficiency Key laboratory, School of Electrical and Electronic Engineering, Huazhong University of Science and Technology, Wuhan 430074, Ch
Liu Y: Department of Electrical Engineering and Computer Science, University of Tennessee, Knoxville, TN 37996, USA
[Login] to see author email addresses.
Journal Name
Energies
Volume
11
Issue
4
Article Number
E705
Year
2018
Publication Date
2018-03-21
Published Version
ISSN
1996-1073
Version Comments
Original Submission
Other Meta
PII: en11040705, Publication Type: Journal Article
Record Map
Published Article
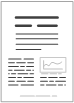
LAPSE:2020.0665
This Record
External Link
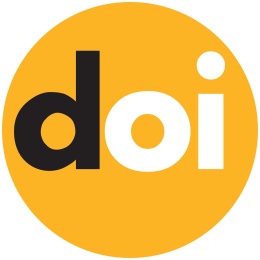
doi:10.3390/en11040705
Publisher Version
Download
Meta
Record Statistics
Record Views
451
Version History
[v1] (Original Submission)
Jun 23, 2020
Verified by curator on
Jun 23, 2020
This Version Number
v1
Citations
Most Recent
This Version
URL Here
https://psecommunity.org/LAPSE:2020.0665
Original Submitter
Calvin Tsay
Links to Related Works