LAPSE:2020.0636
Published Article
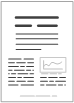
LAPSE:2020.0636
Short-Term Load Forecasting Using Smart Meter Data: A Generalization Analysis
June 23, 2020
Short-term load forecasting ensures the efficient operation of power systems besides affording continuous power supply for energy consumers. Smart meters that are capable of providing detailed information on buildings energy consumption, open several doors of opportunity to short-term load forecasting at the individual building level. In the current paper, four machine learning methods have been employed to forecast the daily peak and hourly energy consumption of domestic buildings. The utilized models depend merely on buildings historical energy consumption and are evaluated on the profiles that were not previously trained on. It is evident that developing data-driven models lacking external information such as weather and building data are of great importance under the situations that the access to such information is limited or the computational procedures are costly. Moreover, the performance evaluation of the models on separated house profiles determines their generalization ability for unseen consumption profiles. The conducted experiments on the smart meter data of several UK houses demonstrated that if the models are fed with sufficient historical data, they can be generalized to a satisfactory level and produce quite accurate results even if they only use past consumption values as the predictor variables. Furthermore, among the four applied models, the ones based on deep learning and ensemble techniques, display better performance in predicting daily peak load consumption than those of others.
Record ID
Keywords
deep learning, generalization analysis, Machine Learning, short-term load forecasting, smart meters
Subject
Suggested Citation
Mehdipour Pirbazari A, Farmanbar M, Chakravorty A, Rong C. Short-Term Load Forecasting Using Smart Meter Data: A Generalization Analysis. (2020). LAPSE:2020.0636
Author Affiliations
Mehdipour Pirbazari A: Department of Electrical and Computer Engineering, University of Stavanger, 4036 Stavanger, Norway
Farmanbar M: Department of Electrical and Computer Engineering, University of Stavanger, 4036 Stavanger, Norway
Chakravorty A: Department of Electrical and Computer Engineering, University of Stavanger, 4036 Stavanger, Norway
Rong C: Department of Electrical and Computer Engineering, University of Stavanger, 4036 Stavanger, Norway
Farmanbar M: Department of Electrical and Computer Engineering, University of Stavanger, 4036 Stavanger, Norway
Chakravorty A: Department of Electrical and Computer Engineering, University of Stavanger, 4036 Stavanger, Norway
Rong C: Department of Electrical and Computer Engineering, University of Stavanger, 4036 Stavanger, Norway
Journal Name
Processes
Volume
8
Issue
4
Article Number
E484
Year
2020
Publication Date
2020-04-21
Published Version
ISSN
2227-9717
Version Comments
Original Submission
Other Meta
PII: pr8040484, Publication Type: Journal Article
Record Map
Published Article
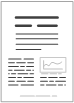
LAPSE:2020.0636
This Record
External Link
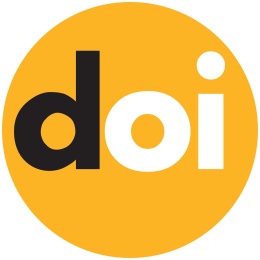
doi:10.3390/pr8040484
Publisher Version
Download
Meta
Record Statistics
Record Views
444
Version History
[v1] (Original Submission)
Jun 23, 2020
Verified by curator on
Jun 23, 2020
This Version Number
v1
Citations
Most Recent
This Version
URL Here
https://psecommunity.org/LAPSE:2020.0636
Original Submitter
Calvin Tsay
Links to Related Works