LAPSE:2019.1316
Published Article
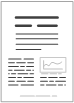
LAPSE:2019.1316
Load State Identification Method for Ball Mills Based on Improved EWT, Multiscale Fuzzy Entropy and AEPSO_PNN Classification
December 10, 2019
To overcome the difficulty of accurately determining the load state of a wet ball mill during the grinding process, a method of mill load identification based on improved empirical wavelet transform (EWT), multiscale fuzzy entropy (MFE), and adaptive evolution particle swarm optimization probabilistic neural network (AEPSO_PNN) classification is proposed. First, the concept of a sliding frequency window is introduced based on EWT, and the adaptive frequency window EWT algorithm, which is used to decompose the vibration signals recorded under different load states to obtain the intrinsic mode components, is proposed. Second, a correlation coefficient threshold is used to select the sensitive mode components that characterize the state of the original signal for signal reconstruction. Finally, the MFE of the reconstructed signal is used as the characteristic vector to characterize the load state of the mill, and the partial mean value of MFE is calculated. The results show that the mean value of MFE under different load states varies. To further identify the load state, a characteristic mill load vector is constructed from the MFE values of the reconstructed signal under different load conditions and is used as the input of the AEPSO_PNN model, which then outputs the predicted ball mill load state. Thus, a load state identification model is established. The feasibility of the method is verified based on grinding experiments. The results show that the overall recognition rate of the proposed method is as high as 97.3%. Compared with the back propagation (BP) neural network, Bayes discriminant method, and PNN classification, AEPSO_PNN classification increases the overall recognition rate by 8%, 5.3%, and 3.3%, respectively, which indicates that this method can be used to accurately identify the different load states of a ball mill.
Record ID
Keywords
EWT, load identification, multiscale fuzzy entropy, PNN
Subject
Suggested Citation
Cai G, Liu X, Dai C, Luo X. Load State Identification Method for Ball Mills Based on Improved EWT, Multiscale Fuzzy Entropy and AEPSO_PNN Classification. (2019). LAPSE:2019.1316
Author Affiliations
Cai G: School of Mechanical and Electrical Engineering, Jiangxi University of Science and Technology, Ganzhou 341000, China
Liu X: School of Mechanical and Electrical Engineering, Jiangxi University of Science and Technology, Ganzhou 341000, China
Dai C: School of Mechanical and Electrical Engineering, Jiangxi University of Science and Technology, Ganzhou 341000, China
Luo X: School of Mechanical and Electrical Engineering, Jiangxi University of Science and Technology, Ganzhou 341000, China
Liu X: School of Mechanical and Electrical Engineering, Jiangxi University of Science and Technology, Ganzhou 341000, China
Dai C: School of Mechanical and Electrical Engineering, Jiangxi University of Science and Technology, Ganzhou 341000, China
Luo X: School of Mechanical and Electrical Engineering, Jiangxi University of Science and Technology, Ganzhou 341000, China
Journal Name
Processes
Volume
7
Issue
10
Article Number
E725
Year
2019
Publication Date
2019-10-11
Published Version
ISSN
2227-9717
Version Comments
Original Submission
Other Meta
PII: pr7100725, Publication Type: Journal Article
Record Map
Published Article
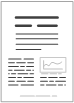
LAPSE:2019.1316
This Record
External Link
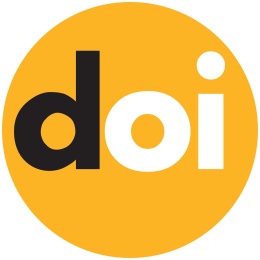
doi:10.3390/pr7100725
Publisher Version
Download
Meta
Record Statistics
Record Views
442
Version History
[v1] (Original Submission)
Dec 10, 2019
Verified by curator on
Dec 10, 2019
This Version Number
v1
Citations
Most Recent
This Version
URL Here
https://psecommunity.org/LAPSE:2019.1316
Original Submitter
Calvin Tsay
Links to Related Works