LAPSE:2019.1203
Published Article
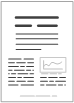
LAPSE:2019.1203
A Comparison of Clustering and Prediction Methods for Identifying Key Chemical−Biological Features Affecting Bioreactor Performance
November 24, 2019
Chemical−biological systems, such as bioreactors, contain stochastic and non-linear interactions which are difficult to characterize. The highly complex interactions between microbial species and communities may not be sufficiently captured using first-principles, stationary, or low-dimensional models. This paper compares and contrasts multiple data analysis strategies, which include three predictive models (random forests, support vector machines, and neural networks), three clustering models (hierarchical, Gaussian mixtures, and Dirichlet mixtures), and two feature selection approaches (mean decrease in accuracy and its conditional variant). These methods not only predict the bioreactor outcome with sufficient accuracy, but the important features correlated with said outcome are also identified. The novelty of this work lies in the extensive exploration and critique of a wide arsenal of methods instead of single methods, as observed in many papers of similar nature. The results show that random forest models predict the test set outcomes with the highest accuracy. The identified contributory features include process features which agree with domain knowledge, as well as several different biomarker operational taxonomic units (OTUs). The results reinforce the notion that both chemical and biological features significantly affect bioreactor performance. However, they also indicate that the quality of the biological features can be improved by considering non-clustering methods, which may better represent the true behaviour within the OTU communities.
Record ID
Keywords
bioinformatics, Machine Learning, statistics
Subject
Suggested Citation
Tsai Y, Baldwin SA, Siang LC, Gopaluni B. A Comparison of Clustering and Prediction Methods for Identifying Key Chemical−Biological Features Affecting Bioreactor Performance. (2019). LAPSE:2019.1203
Author Affiliations
Tsai Y: Department of Chemical and Biological Engineering, University of British Columbia, Vancouver, BC V6T 1Z3, Canada
Baldwin SA: Department of Chemical and Biological Engineering, University of British Columbia, Vancouver, BC V6T 1Z3, Canada
Siang LC: Department of Chemical and Biological Engineering, University of British Columbia, Vancouver, BC V6T 1Z3, Canada [ORCID]
Gopaluni B: Department of Chemical and Biological Engineering, University of British Columbia, Vancouver, BC V6T 1Z3, Canada [ORCID]
[Login] to see author email addresses.
Baldwin SA: Department of Chemical and Biological Engineering, University of British Columbia, Vancouver, BC V6T 1Z3, Canada
Siang LC: Department of Chemical and Biological Engineering, University of British Columbia, Vancouver, BC V6T 1Z3, Canada [ORCID]
Gopaluni B: Department of Chemical and Biological Engineering, University of British Columbia, Vancouver, BC V6T 1Z3, Canada [ORCID]
[Login] to see author email addresses.
Journal Name
Processes
Volume
7
Issue
9
Article Number
E614
Year
2019
Publication Date
2019-09-10
Published Version
ISSN
2227-9717
Version Comments
Original Submission
Other Meta
PII: pr7090614, Publication Type: Journal Article
Record Map
Published Article
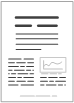
LAPSE:2019.1203
This Record
External Link
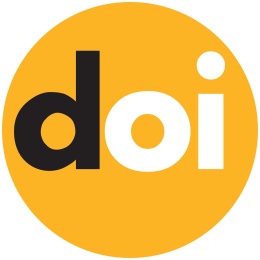
doi:10.3390/pr7090614
Publisher Version
Download
Meta
Record Statistics
Record Views
595
Version History
[v1] (Original Submission)
Nov 24, 2019
Verified by curator on
Nov 24, 2019
This Version Number
v1
Citations
Most Recent
This Version
URL Here
https://psecommunity.org/LAPSE:2019.1203
Original Submitter
Calvin Tsay
Links to Related Works