LAPSE:2019.1053
Published Article
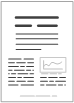
LAPSE:2019.1053
Research on Green Management Effect Evaluation of Power Generation Enterprises in China Based on Dynamic Hesitation and Improved Extreme Learning Machine
September 23, 2019
Carbon emissions and environmental protection issues have become the pressure from the international community during the current transitional stage of China’s energy transformation. China has set a macro carbon emission target, which will reduce carbon emissions per unit of Gross Domestic Product (GDP) by 40% in 2020 and 60−65% in 2030 than that in 2005. To achieve the emission reduction target, the industrial structure must be adjusted and upgraded. Furthermore, it must start from a high-pollution and high-emission industry. Therefore, it is of practical significance to construct a low-carbon sustainability and green operation benefits of power generation enterprises to save energy and reduce emissions. In this paper, an intuitionistic fuzzy comprehensive analytic hierarchy process based on improved dynamic hesitation degree (D-IFAHP) and an improved extreme learning machine algorithm optimized by RBF kernel function (RELM) are proposed. Firstly, we construct the evaluation indicator system of low-carbon sustainability and green operation benefits of power generation enterprises. Moreover, during the non-dimensional processing, the evaluation index system is determined. Secondly, we apply the evaluation indicator system by an empirical analysis. It is proved that the D-IFAHP evaluation model proposed in this paper has higher accuracy performance. Finally, the RELM is applied to D-IFAHP to construct a combined evaluation model named D-IFAHP-RELM evaluation model. The D-IFAHP evaluation results are used as the input of the training sets of the RELM algorithm, which simplifies the comprehensive evaluation process and can be directly applied to similar projects.
Record ID
Keywords
dynamic hesitation, evaluation index system for power generation enterprises, improved extreme learning machine, intuitionistic fuzzy analytic hierarchy process, low-carbon sustainability and green operation benefits
Subject
Suggested Citation
Qin Y, Li M, De G, Huang L, Yang S, Tan Q, Tan Z, Zhou F. Research on Green Management Effect Evaluation of Power Generation Enterprises in China Based on Dynamic Hesitation and Improved Extreme Learning Machine. (2019). LAPSE:2019.1053
Author Affiliations
Qin Y: School of Economics and Management, North China Electric Power University, Beijing 102206, China
Li M: School of Economics and Management, North China Electric Power University, Beijing 102206, China; Beijing Key Laboratory of New Energy and Low-Carbon Development, North China Electric Power University, Beijing 102206, China
De G: School of Economics and Management, North China Electric Power University, Beijing 102206, China; Beijing Key Laboratory of New Energy and Low-Carbon Development, North China Electric Power University, Beijing 102206, China
Huang L: School of Economics and Management, North China Electric Power University, Beijing 102206, China; Beijing Key Laboratory of New Energy and Low-Carbon Development, North China Electric Power University, Beijing 102206, China
Yang S: School of Economics and Management, North China Electric Power University, Beijing 102206, China; Beijing Key Laboratory of New Energy and Low-Carbon Development, North China Electric Power University, Beijing 102206, China
Tan Q: State Grid Energy Research Institute Co., LTD., Beijing 102209, China [ORCID]
Tan Z: School of Economics and Management, North China Electric Power University, Beijing 102206, China; Beijing Key Laboratory of New Energy and Low-Carbon Development, North China Electric Power University, Beijing 102206, China; School of Economics and Manage
Zhou F: School of Economics and Management, North China Electric Power University, Beijing 102206, China
[Login] to see author email addresses.
Li M: School of Economics and Management, North China Electric Power University, Beijing 102206, China; Beijing Key Laboratory of New Energy and Low-Carbon Development, North China Electric Power University, Beijing 102206, China
De G: School of Economics and Management, North China Electric Power University, Beijing 102206, China; Beijing Key Laboratory of New Energy and Low-Carbon Development, North China Electric Power University, Beijing 102206, China
Huang L: School of Economics and Management, North China Electric Power University, Beijing 102206, China; Beijing Key Laboratory of New Energy and Low-Carbon Development, North China Electric Power University, Beijing 102206, China
Yang S: School of Economics and Management, North China Electric Power University, Beijing 102206, China; Beijing Key Laboratory of New Energy and Low-Carbon Development, North China Electric Power University, Beijing 102206, China
Tan Q: State Grid Energy Research Institute Co., LTD., Beijing 102209, China [ORCID]
Tan Z: School of Economics and Management, North China Electric Power University, Beijing 102206, China; Beijing Key Laboratory of New Energy and Low-Carbon Development, North China Electric Power University, Beijing 102206, China; School of Economics and Manage
Zhou F: School of Economics and Management, North China Electric Power University, Beijing 102206, China
[Login] to see author email addresses.
Journal Name
Processes
Volume
7
Issue
7
Article Number
E474
Year
2019
Publication Date
2019-07-22
Published Version
ISSN
2227-9717
Version Comments
Original Submission
Other Meta
PII: pr7070474, Publication Type: Journal Article
Record Map
Published Article
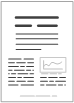
LAPSE:2019.1053
This Record
External Link
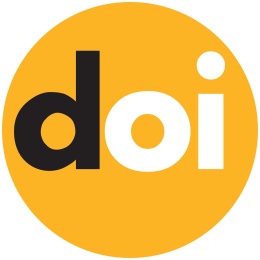
doi:10.3390/pr7070474
Publisher Version
Download
Meta
Record Statistics
Record Views
531
Version History
[v1] (Original Submission)
Sep 23, 2019
Verified by curator on
Sep 23, 2019
This Version Number
v1
Citations
Most Recent
This Version
URL Here
https://psecommunity.org/LAPSE:2019.1053
Original Submitter
Calvin Tsay
Links to Related Works