LAPSE:2019.0897
Published Article
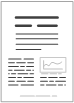
LAPSE:2019.0897
Day-Ahead Prediction of Microgrid Electricity Demand Using a Hybrid Artificial Intelligence Model
August 5, 2019
Improved-performance day-ahead electricity demand forecast is important to deliver necessary information for right decision of energy management of microgrids. It supports microgrid operators and stakeholders to have better decisions on microgrid flexibility, stability and control. The available conventional forecasting methods for electricity demand at national or regional level are not effective for electricity demand forecasting in microgrids. This is due to the fact that the electricity consumption in microgrids is many times less than the regional or national demands and it is highly volatile. In this paper, an integrated Artificial Intelligence (AI) based approach consisting of Wavelet Transform (WT), Simulated Annealing (SA) and Feedforward Artificial Neural Network (FFANN) is devised for day-ahead prediction of electric power consumption in microgrids. The FFANN is the basic forecasting engine of the proposed model. The WT is utilized to extract relevant features of the target variable (electric load data series) to obtain a cluster of enhanced-feature subseries. The extracted subseries of the past values of the electric load demand data are employed as the target variables to model the FFANN. The SA optimization technique is employed to obtain the optimal values of the FFANN weight parameters during the training process. Historical information of actual electricity consumption, meteorological variables, daily variations, weekly variations, and working/non-working day indicators have been employed to develop the forecasting tool of the devised integrated AI based approach. The approach is validated using electricity demand data of an operational microgrid in Beijing, China. The prediction results are presented for future testing days with one-hour time interval. The validation results demonstrated that the devised approach is capable to forecast the microgrid electricity demand with acceptably small error and reasonably short computation time. Moreover, the prediction performance of the devised approach has been evaluated relative to other four approaches and resulted in better prediction accuracy.
Record ID
Keywords
Artificial Intelligence, electricity demand, feedforward artificial neural network, forecasting, microgrid, simulated annealing, smart grid, wavelet transform
Subject
Suggested Citation
Ma YJ, Zhai MY. Day-Ahead Prediction of Microgrid Electricity Demand Using a Hybrid Artificial Intelligence Model. (2019). LAPSE:2019.0897
Author Affiliations
Ma YJ: School of Electronic Information Engineering, Guangdong University of Petrochemical Technology, No. 139 Guandu Road, Maoming 525000, China
Zhai MY: School of Electronic Information Engineering, Guangdong University of Petrochemical Technology, No. 139 Guandu Road, Maoming 525000, China
[Login] to see author email addresses.
Zhai MY: School of Electronic Information Engineering, Guangdong University of Petrochemical Technology, No. 139 Guandu Road, Maoming 525000, China
[Login] to see author email addresses.
Journal Name
Processes
Volume
7
Issue
6
Article Number
E320
Year
2019
Publication Date
2019-05-30
Published Version
ISSN
2227-9717
Version Comments
Original Submission
Other Meta
PII: pr7060320, Publication Type: Journal Article
Record Map
Published Article
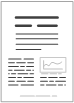
LAPSE:2019.0897
This Record
External Link
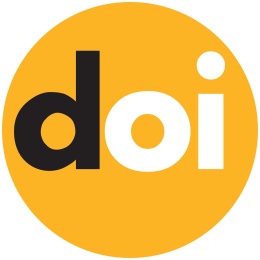
doi:10.3390/pr7060320
Publisher Version
Download
Meta
Record Statistics
Record Views
572
Version History
[v1] (Original Submission)
Aug 5, 2019
Verified by curator on
Aug 5, 2019
This Version Number
v1
Citations
Most Recent
This Version
URL Here
https://psecommunity.org/LAPSE:2019.0897
Original Submitter
Calvin Tsay
Links to Related Works