LAPSE:2019.0829
Published Article
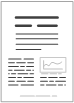
LAPSE:2019.0829
DA-Based Parameter Optimization of Combined Kernel Support Vector Machine for Cancer Diagnosis
July 29, 2019
As is well known, the correct diagnosis for cancer is critical to save patients’ lives. Support vector machine (SVM) has already made an important contribution to the field of cancer classification. However, different kernel function configurations and their parameters will significantly affect the performance of SVM classifier. To improve the classification accuracy of SVM classifier for cancer diagnosis, this paper proposed a novel cancer classification algorithm based on the dragonfly algorithm and SVM with a combined kernel function (DA-CKSVM) which was constructed from a radial basis function (RBF) kernel and a polynomial kernel. Experiments were performed on six cancer data sets from University of California, Irvine (UCI) machine learning repository and two cancer data sets from Cancer Program Legacy Publication Resources to evaluate the validity of the proposed algorithm. Compared with four well-known algorithms: dragonfly algorithm-SVM (DA-SVM), particle swarm optimization-SVM (PSO-SVM), bat algorithm-SVM (BA-SVM), and genetic algorithm-SVM (GA-SVM), the proposed algorithm was able to find the optimal parameters of SVM classifier and achieved better classification accuracy on cancer datasets.
Record ID
Keywords
cancer, classification, combined kernel function, dragonfly algorithm, parameter optimization, support vector machine (SVM)
Subject
Suggested Citation
Xie T, Yao J, Zhou Z. DA-Based Parameter Optimization of Combined Kernel Support Vector Machine for Cancer Diagnosis. (2019). LAPSE:2019.0829
Author Affiliations
Xie T: School of Mechatronic Engineering and Automation, Shanghai University, 99 ShangDa Road, Shanghai 200444, China
Yao J: School of Mechatronic Engineering and Automation, Shanghai University, 99 ShangDa Road, Shanghai 200444, China
Zhou Z: School of Mechatronic Engineering and Automation, Shanghai University, 99 ShangDa Road, Shanghai 200444, China
[Login] to see author email addresses.
Yao J: School of Mechatronic Engineering and Automation, Shanghai University, 99 ShangDa Road, Shanghai 200444, China
Zhou Z: School of Mechatronic Engineering and Automation, Shanghai University, 99 ShangDa Road, Shanghai 200444, China
[Login] to see author email addresses.
Journal Name
Processes
Volume
7
Issue
5
Article Number
E263
Year
2019
Publication Date
2019-05-06
Published Version
ISSN
2227-9717
Version Comments
Original Submission
Other Meta
PII: pr7050263, Publication Type: Journal Article
Record Map
Published Article
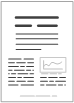
LAPSE:2019.0829
This Record
External Link
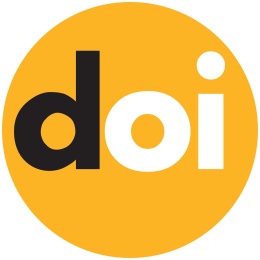
doi:10.3390/pr7050263
Publisher Version
Download
Meta
Record Statistics
Record Views
410
Version History
[v1] (Original Submission)
Jul 29, 2019
Verified by curator on
Jul 29, 2019
This Version Number
v1
Citations
Most Recent
This Version
URL Here
https://psecommunity.org/LAPSE:2019.0829
Original Submitter
Calvin Tsay
Links to Related Works