LAPSE:2018.1132
Published Article
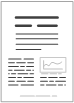
LAPSE:2018.1132
Multi-Objective Sustainable Operation of the Three Gorges Cascaded Hydropower System Using Multi-Swarm Comprehensive Learning Particle Swarm Optimization
November 28, 2018
Optimal operation of hydropower reservoir systems often needs to optimize multiple conflicting objectives simultaneously. The conflicting objectives result in a Pareto front, which is a set of non-dominated solutions. Non-dominated solutions cannot outperform each other on all the objectives. An optimization framework based on the multi-swarm comprehensive learning particle swarm optimization algorithm is proposed to solve the multi-objective operation of hydropower reservoir systems. Through adopting search techniques such as decomposition, mutation and differential evolution, the algorithm tries to derive multiple non-dominated solutions reasonably distributed over the true Pareto front in one single run, thereby facilitating determining the final tradeoff. The long-term sustainable planning of the Three Gorges cascaded hydropower system consisting of the Three Gorges Dam and Gezhouba Dam located on the Yangtze River in China is studied. Two conflicting objectives, i.e., maximizing hydropower generation and minimizing deviation from the outflow lower target to realize the system’s economic, environmental and social benefits during the drought season, are optimized simultaneously. Experimental results demonstrate that the optimization framework helps to robustly derive multiple feasible non-dominated solutions with satisfactory convergence, diversity and extremity in one single run for the case studied.
Record ID
Keywords
comprehensive learning, hydropower reservoir system, multi-objective optimal operation, multi-swarm, Particle Swarm Optimization
Subject
Suggested Citation
Yu X, Sun H, Wang H, Liu Z, Zhao J, Zhou T, Qin H. Multi-Objective Sustainable Operation of the Three Gorges Cascaded Hydropower System Using Multi-Swarm Comprehensive Learning Particle Swarm Optimization. (2018). LAPSE:2018.1132
Author Affiliations
Yu X: Provincial Key Laboratory for Water Information Cooperative Sensing and Intelligent Processing, Nanchang Institute of Technology, 289 Tianxiang Road, Nanchang 330099, Jiangxi, China
Sun H: Provincial Key Laboratory for Water Information Cooperative Sensing and Intelligent Processing, Nanchang Institute of Technology, 289 Tianxiang Road, Nanchang 330099, Jiangxi, China
Wang H: Provincial Key Laboratory for Water Information Cooperative Sensing and Intelligent Processing, Nanchang Institute of Technology, 289 Tianxiang Road, Nanchang 330099, Jiangxi, China
Liu Z: Provincial Key Laboratory for Water Information Cooperative Sensing and Intelligent Processing, Nanchang Institute of Technology, 289 Tianxiang Road, Nanchang 330099, Jiangxi, China
Zhao J: Provincial Key Laboratory for Water Information Cooperative Sensing and Intelligent Processing, Nanchang Institute of Technology, 289 Tianxiang Road, Nanchang 330099, Jiangxi, China
Zhou T: Economic Research Institute, State Grid Jiangxi Power Corporation, 1588 Yingbinbei Road, Nanchang 330043, Jiangxi, China [ORCID]
Qin H: School of Hydropower and Information Engineering, Huazhong University of Science and Technology, 1037 Luoyu Road, Wuhan 430074, Hubei, China
[Login] to see author email addresses.
Sun H: Provincial Key Laboratory for Water Information Cooperative Sensing and Intelligent Processing, Nanchang Institute of Technology, 289 Tianxiang Road, Nanchang 330099, Jiangxi, China
Wang H: Provincial Key Laboratory for Water Information Cooperative Sensing and Intelligent Processing, Nanchang Institute of Technology, 289 Tianxiang Road, Nanchang 330099, Jiangxi, China
Liu Z: Provincial Key Laboratory for Water Information Cooperative Sensing and Intelligent Processing, Nanchang Institute of Technology, 289 Tianxiang Road, Nanchang 330099, Jiangxi, China
Zhao J: Provincial Key Laboratory for Water Information Cooperative Sensing and Intelligent Processing, Nanchang Institute of Technology, 289 Tianxiang Road, Nanchang 330099, Jiangxi, China
Zhou T: Economic Research Institute, State Grid Jiangxi Power Corporation, 1588 Yingbinbei Road, Nanchang 330043, Jiangxi, China [ORCID]
Qin H: School of Hydropower and Information Engineering, Huazhong University of Science and Technology, 1037 Luoyu Road, Wuhan 430074, Hubei, China
[Login] to see author email addresses.
Journal Name
Energies
Volume
9
Issue
6
Article Number
E438
Year
2016
Publication Date
2016-06-07
Published Version
ISSN
1996-1073
Version Comments
Original Submission
Other Meta
PII: en9060438, Publication Type: Journal Article
Record Map
Published Article
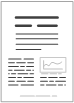
LAPSE:2018.1132
This Record
External Link
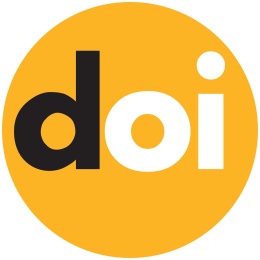
doi:10.3390/en9060438
Publisher Version
Download
Meta
Record Statistics
Record Views
625
Version History
[v1] (Original Submission)
Nov 28, 2018
Verified by curator on
Nov 28, 2018
This Version Number
v1
Citations
Most Recent
This Version
URL Here
https://psecommunity.org/LAPSE:2018.1132
Original Submitter
Calvin Tsay
Links to Related Works